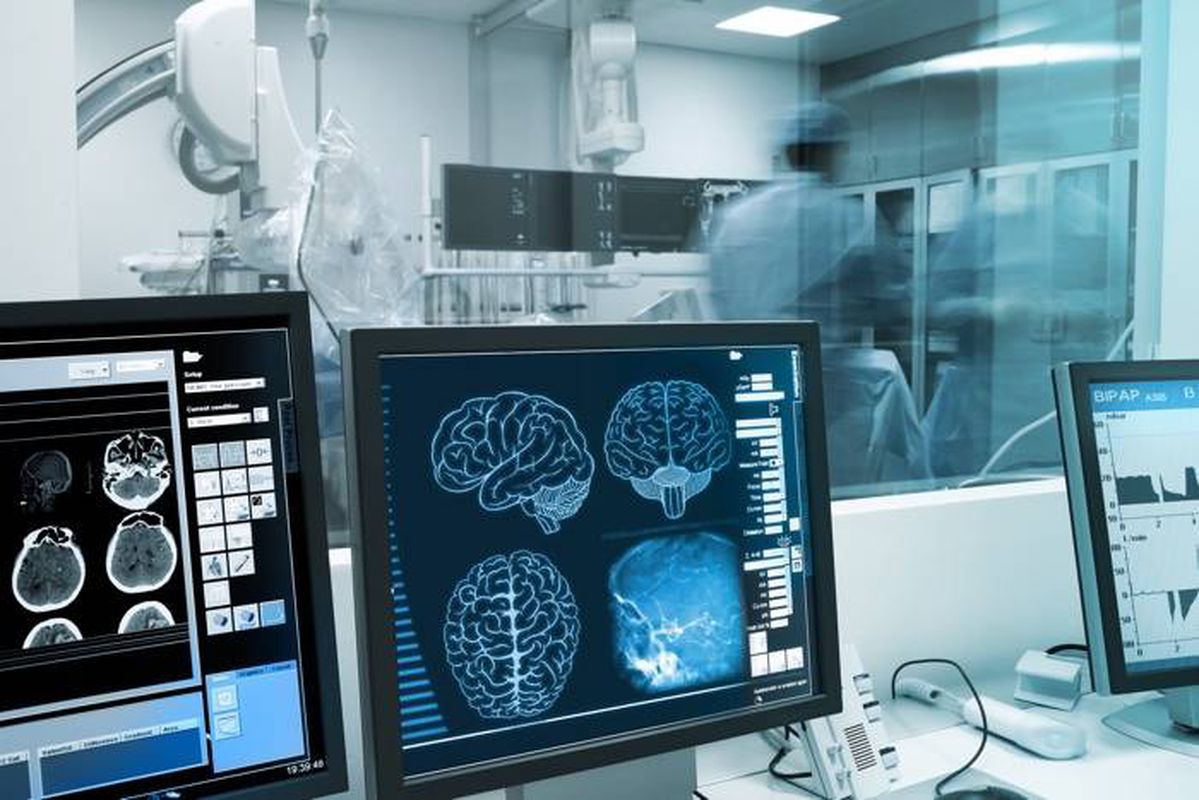
Having a high-quality source of labeled medical data is a key condition for the successful creation of AI solutions for healthcare. This is claimed by serious analytical studies such as the «Artificial Intelligence for Health and Health Care» https://www.healthit.gov/ (we have published our translation to Russian here) and “THINKING ON ITS OWN: AI IN THE NHS” https://reform.uk/ (we have published the translation to Russian here). What is more, numerous popular publications in the media and blogs discuss this as well.
In fact, a good feasible idea and prepared data are the two main prerequisites for creating an artificial intelligence system. And if they are absent, perhaps these are the main reasons for the failure of such a project.
It is well known that the analysis of medical images and the creation of clinical decision support systems in diagnostics is one of the most popular and currently developing areas of application of artificial intelligence. To support such developments, we publish a brief overview of the most prominent open source medical imaging data sets that can be found on the Internet. At the same time, we have to say that this list is provided for informational purposes only. Before using these databases, make sure you understand and comply with the restrictions imposed by their owners.
OmniMedicalSearh database. Huge database of various data from medical sources such as an interactive anatomy atlas, a variety of medical image collections, dermatological tests results, a library of endoscopic videos. Access: http://www.omnimedicalsearch.com/image_databases.html
The National Library of Medicine MedPix. The database contains 53 thousand medical images of 13 thousand patients with annotations. Registration is required. Link: https://medpix.nlm.nih.gov/home
MURA X-ray database (musculoskeletal radiographs). It is a musculoskeletal X-ray dataset which consists of 14,863 examinations from 12,173 patients, for a total of 40,561 reusable radiographic images. Each refers to one of the 7 standard types of x-rays of the upper limb: elbow, finger, forearm, arm, humerus, shoulder, and wrist. Each study was manually labeled as normal or abnormal by board-certified radiologists at Stanford Hospital between 2001 and 2012. Description: https://stanfordmlgroup.github.io/competitions/mura/
ABIDE System (The Autism Brain Imaging Data Exchange). Function MRI images for 539 individuals suffering from ASD and 573 typical controls. These 1112 datasets are composed of structural and resting state functional MRI data along with an extensive array of phenotypic information. Registration is required. Description: http://www.ncbi.nlm.nih.gov/pubmed/23774715. Preprocessed version: http://preprocessed-connectomes-project.org/abide/
Alzheimer's Disease Neuroimaging Initiative - ADNI. MRI database on Alzheimer's patients and healthy controls. Also has clinical, genomic, and biomaker data. Requires registration. Description: http://www.neurology.org/content/74/3/201.short. Access: http://adni.loni.usc.edu/data-samples/access-data/
Digital Retinal Images for Vessel Extraction - DRIVE. The DRIVE database is for comparative studies on segmentation of blood vessels in retinal images. It consists of photographs that show signs of mild early diabetic retinopathy. Description: http://www.isi.uu.nl/Research/Publications/publicationview/id=855.html. Access: http://www.isi.uu.nl/Research/Databases/DRIVE/download.php
The Open Access Series of Imaging Studies - OASIS. Two datasets are available: a cross-sectional and a longitudinal set. Access: http://www.oasis-brains.org/
SCMR Consensus Data. The SCMR Consensus Dataset is a set of 15 cardiac MRI studies of mixed pathologies (5 healthy, 6 myocardial infarction, 2 heart failure and 2 hypertrophy), which were acquired from different MR machines (4 GE, 5 Siemens, 6 Philips). Access: http://www.cardiacatlas.org/studies/
Lung Image Database Consortium - LIDC. Preliminary clinical studies have shown that spiral CT scanning of the lungs can improve early detection of lung cancer in high-risk individuals. Image processing algorithms have the potential to assist in lesion detection on spiral CT studies, and to assess the stability or change in lesion size on serial CT studies. The use of such computer-assisted algorithms could significantly enhance the sensitivity and specificity of spiral CT lung screening, as well as lower costs by reducing physician time needed for interpretation. Access: http://imaging.cancer.gov/programsandresources/informationsystems/lidc
NIH Chest X-ray Dataset of 14 Common Thorax Disease Categories. The dataset contains 112,000 images of 30,000 unique patients with a frontal view and examples of 14 thoracic pathologies. Access: http://academictorrents.com/details/557481faacd824c83fbf57dcf7b6da9383b3235a
The Cancer Imaging Archive (TCIA) Collections. Cancer imaging data sets across various cancer types (e.g. carcinoma, lung cancer, myeloma) and various imaging modalities. The image data in The Cancer Imaging Archive (TCIA) is organized into purpose-built collections of subjects. The subjects typically have a cancer type and/or anatomical site (lung, brain, etc.) in common. Each link in the table below contains information concerning the scientific value of a collection, information about how to obtain any supporting non-image data which may be available, and links to view or download the imaging data. To support reproducibility in scientific research, TCIA supports Digital Object Identifiers (DOIs) which allow users to share subsets of TCIA data referenced in a research manuscript. Access: http://www.cancerimagingarchive.net/
Belarus tuberculosis portal. Tuberculosis (TB) is a major problem of Belarus Public Health. Many and the most severe cases usually disseminate across the country to different TB dispensaries. The ability of leading Belarus TB specialists to follow such patients will be greatly improved by using a common database containing patients’ radiological images, lab work and clinical data. This will also significantly improve adherence to the treatment protocol and result in a better record of the treatment outcomes. Belarus dataset have both chest X-rays and CT scans of the same patient. Access: http://tuberculosis.by/
The Digital Database for Screening Mammography (DDSM) is a resource for use by the mammographic image analysis research community. Primary support for this project was a grant from the Breast Cancer Research Program of the U.S. Army Medical Research and Materiel Command. The DDSM project is a collaborative effort involving co-p.i.s at the Massachusetts General Hospital (D. Kopans, R. Moore), the University of South Florida (K. Bowyer), and Sandia National Laboratories (P. Kegelmeyer). Additional cases from Washington University School of Medicine were provided by Peter E. Shile, MD, Assistant Professor of Radiology and Internal Medicine. Additional collaborating institutions include Wake Forest University School of Medicine (Departments of Medical Engineering and Radiology), Sacred Heart Hospital and ISMD, Incorporated. The primary purpose of the database is to facilitate sound research in the development of computer algorithms to aid in screening. Secondary purposes of the database may include the development of algorithms to aid in the diagnosis and the development of teaching or training aids. The database contains approximately 2,500 studies. Each study includes two images of each breast, along with some associated patient information (age at time of study, ACR breast density rating, subtlety rating for abnormalities, ACR keyword description of abnormalities) and image information (scanner, spatial resolution, ...). Images containing suspicious areas have associated pixel-level "ground truth" information about the locations and types of suspicious regions. Also provided are software both for accessing the mammogram and truth images and for calculating performance figures for automated image analysis algorithms. Access: http://marathon.csee.usf.edu/Mammography/Database.html
Database of MRI images of prostate cancer. Magnetic resonance imaging (MRI) provides imaging techniques allowing diagnosing and localizing CaP. The I2CVB provides a multi-parametric MRI dataset to help at the development of computer-aided detection and diagnosis (CAD) system. Access: http://i2cvb.github.io/
Segmentation in Chest Radiographs – SCR. The automatic segmentation of anatomical structures in chest radiographs is of great importance for computer-aided diagnosis in these images. The SCR database has been established to facilitate comparative studies on segmentation of the lung fields, the heart and the clavicles in standard posterior-anterior chest radiographs. Access: http://www.isi.uu.nl/Research/Databases/SCR/
VIA Group Public Databases. Includes documented image databases suitable for the development of quantitative image analysis tools, especially in clinical decision support systems (CDDS).It is established in collaboration with the I-ELCAP group and contains lung CT images in the DICOM format together with documentation of abnormalities by radiologists. Access: http://www.via.cornell.edu/databases/
The USC-SIPI Image Database is a collection of digitized images. It is maintained primarily to support research in image processing, image analysis, and machine vision. The first edition of the USC-SIPI image database was distributed in 1977 and many new images have been added since then. The database is divided into volumes based on the basic character of the pictures. Images in each volume are of various sizes such as 256x256 pixels, 512x512 pixels, or 1024x1024 pixels. All images are 8 bits/pixel for black and white images, 24 bits/pixel for color images. Access: http://sipi.usc.edu/database/
Visual Concept Extraction Challenge in Radiology. Manually annotated radiological data of several anatomical structures (e.g. kidney, lung, bladder, etc.) from several different imaging modalities (e.g. CT and MR). They also provide a cloud computing instance that anyone can use to develop and evaluate models against benchmarks. Access: http://www.visceral.eu/
Kaggle diabetic retinopathy. High-resolution retinal images that are annotated on a 0–4 severity scale by clinicians, for the detection of diabetic retinopathy. This data set is part of a completed Kaggle competition, which is generally a great source for publicly available data sets. Access: https://www.kaggle.com/c/diabetic-retinopathy-detection
Cervical Cancer Screening. Another source of data from the Kaggle competition, this time used in developing algorithms to correctly classify cervical types based on the corresponding images. These different types of cervix in our data set are all considered normal (not cancerous), but since the transformation zones aren't always visible, some of the patients require further testing while some don't. один источник данных конкурсов kaggle, на этот раз использовался при разработке алгоритмов для правильной классификации типов шейки матки на основе соответствующих изображений Access: https://www.kaggle.com/c/intel-mobileodt-cervical-cancer-screening/data
Multimodal Brain Tumor Segmentation Challenge. Large data set of brain tumor magnetic resonance scans. The authors been extending this data set and challenge each year since 2012. Access: http://braintumorsegmentation.org/