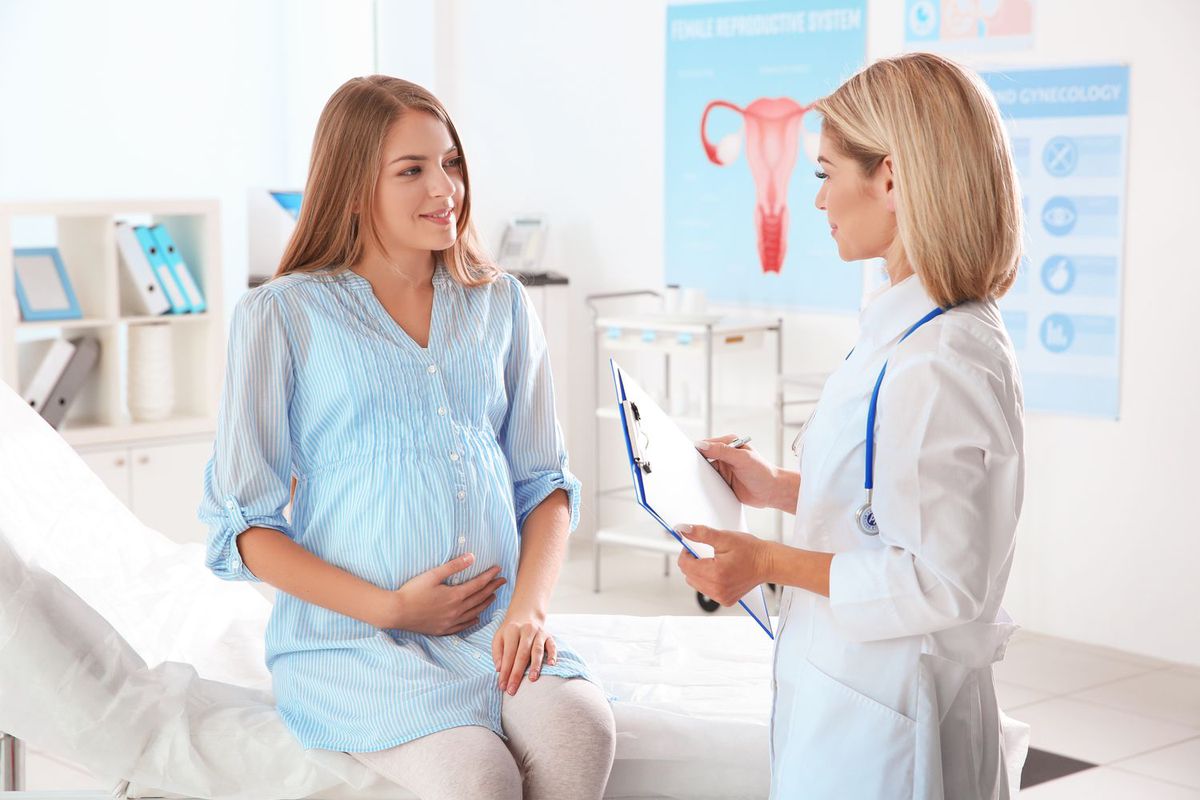
Currently, there is an increased interest in the application of artificial intelligence (AI) technologies in various fields of medicine and health care, including obstetrics and gynecology. The sGynecology, Obstetrics and Perinatology journal has published Artificial Intelligence: Predictive Analytics of Perinatal Risk article, which presents the use of AI in perinatology, indicates the potential limitations of intelligent technologies in medicine and outlines the vector of AI development in assessing perinatal risks.
Advances in machine learning, the availability of large datasets and the increase in computing power are actively driving a rise in interest in artificial intelligence technologies. Machine learning (ML), a kind of AI, allows you to automatically generate mathematical models on large datasets and use them to solve urgent problems, including predicting various events in obstetrics and neonatology. Further integration of artificial intelligence into perinatology will contribute to the development of this area of medicine.
The ability to effectively train on a large number of complex samples and to cope well with the processing of medical data is the advantage of machine learning, incl. deep learning (DL). The accumulation of information from medical, laboratory and diagnostic information systems and their structuring using natural language processing (NLP) allows the formation of large-scale labeled reproductive health data sets. With the help of the complex application of ML and other methods of intellectual analysis, researchers create developed models for working out the results of predicting, diagnosing and treating complications of pregnancy and preventing unfavorable perinatal outcomes. One of the promising areas is the improved personalized treatment of patients using machine learning (Siristatidis C et al. 2016). Patients with unfavorable obstetric and gynecological history can be personally treated with the most appropriate therapy, which increases the success of the onset and gestation of pregnancy and reduces the financial burden of medical costs (Senders JT et al. 2018). With the help of ML, doctors are more and more successful in predicting and diagnosing obstetric pathology, but the main disadvantage of ML is still the need to train models on extremely large data sets. In addition, researchers can only control the input data and individual parameters of the model, so the black-box model is devoid of interpretability (Ching T et al. 2018).
Deep learning demonstrates the great potential and continues to actively develop in the field of perinatal medicine, including the analysis of diagnostic data (Miotto R et al. 2018). Lei B et al. (Lei B et al. 2014) automated the determination of standardized ultrasound scan planes when examining fetal facial structures using an SVM classifier. In 2017, Yu Z et al. (Yu Z et al. 2017) used the potential of deep convolutional neural networks (CNNs) to automatically recognize standardized fetal facial planes during routine ultrasound examinations. Likewise, Rahmatullah B et al. (Rahmatullah B et al. 2011, 2012) trained an adaptive boosting model (AdaBoost) to detect these structures in 2D ultrasound images in order to evaluate image quality. An even more ambitious project using CNN aimed at classifying a wide range of fetal imaging planes by automatically recognizing 14 fetal structures in 2-D ultrasound images (Sridar P et al. 2019). Raynaud C et al. (Raynaud C et al. 2017) proposed DL for feature extraction and RF for classification of organ anatomy in 3D ultrasound scans to automatically encode changes in anatomical structures. The next step towards the automation of fetal ultrasound scanning is to combine the recognition of the planes of fetal images with a robotic arm performing the scan (Wang S et al. 2019).
Accurate estimates of fetal weight and gestational age are essential for identifying any abnormal fetal growth pattern. In 2019, Kim HP et al. published the DL model for the automatic measurement of head circumference and biparietal size from 2D ultrasound images (Kim HP et al. 2019). Li J et al. used a different approach that first used RF to locate the fetal head and then used ellipse fit to estimate head circumference from 2D ultrasound images (Li J et al. 2018). Van den Heuvel TL et al. introduced a DL model that calculated head circumference from obstetric protocol data (van den Heuvel TL et al. 2019). These data most likely did not contain information on standard planes, and therefore their method has great potential for use in countries with limited resources, where there is a shortage of qualified doctors in the field of prenatal diagnosis. In recent decades, AI methods have been proposed to improve the estimation of the gestational age of the fetus in women with uncertain or unknown periods of menstruation (Papageorghiou AT et al. 2016) and to refine the weight of the fetus during pregnancy. For example, Naimi AI et al. tested the hypothesis that birth data can be used to accurately predict estimated fetal weight during pregnancy using various AI techniques such as RF or regression trees in a database of more than 10,000 normal and high-risk pregnancies (Naimi AI et al. 2018). The authors found that AI algorithms estimate fetal weight better than other commonly used methods. Chuang L et al. developed an ANN to estimate fetal weight using morphometric data from 991 fetuses, reporting a mean absolute error of 6.15% (Chuang L et al. 2002).
In addition to fetometry, assessment of fetal weight and gestational age, AI methods were directed by researchers to assess fetal structures and organs in order to detect fetal abnormalities in a timely manner and take the necessary measures. Namburete AI et al. used a radiofrequency classifier to segment cranial pixels in 2-D ultrasound images (Namburete AI et al. 2013). In 2017, Li Y et al. used the DL approach for automatic ultrasound segmentation of the fetal body and amniotic fluid based on 2D scanning data (Li Y et al. 2017). Other examples of DL for segmentation have targeted the fetal brain and lungs (Rajchl M et al. 2017, Burgos-Artizzu XP et al. 2019); and indicated organs and systems, placenta and kidneys of the mother with magnetic resonance imaging (Wang G et al. 2018). Finally, an ensemble of decision trees was used to automatically segment fetal brain structures in 3D ultrasound images (Wang G et al. 2018). For more information on biometric measurements, we refer the reader to a recent review of automated methods for detecting fetal abnormalities (Rawat V et al. 2018).
Thus, the available publications clearly demonstrate that recently AI in obstetrics and perinatology has been used mainly as a predictive analytics tool to improve the efficiency of prenatal diagnosis and assess the risk of adverse outcomes. In most studies, these models are few in number, single source and retrospective. There is still a lack of large-scale randomized controlled trials for external validation of models and optimization of the use of limited research resources. At the same time, most of the research is limited to the use of classification and forecasting algorithms without integrating the obtained analysis data. One of the biggest downsides to deep machine learning is the black-box model, which means that we do not know how the results are generated within the model.
In clinical practice, it is not enough to obtain accurate results, they need to be explained, given that research on the implementation of AI technologies in medicine is often associated with making responsible decisions and treating patients. However, many papers ignore the interpretation of the model, despite the ambiguity of the conclusions. It is important to ensure that models are understandable because good interpretability is critical for clinical applications and is an essential element for successful patient-clinician interactions. In our opinion, more research is needed in the fields of diagnostics, prevention and personalized treatment all based on a remote medical expert automatic AI-associated system.
Reference: Ivshin A.A., Gusev A.V., Novitskiy R.E. Artificial intelligence: predictive analytics of perinatal risks. Vopr. ginekol. akus. perinatol. (Gynecology, Obstetrics and Perinatology). 2020; 19(6): 133–144. (In Russian). DOI: 10.20953/1726-1678-2020-6-133-144. https://doi.org/10.20953/1726-1678-2020-6-133-144