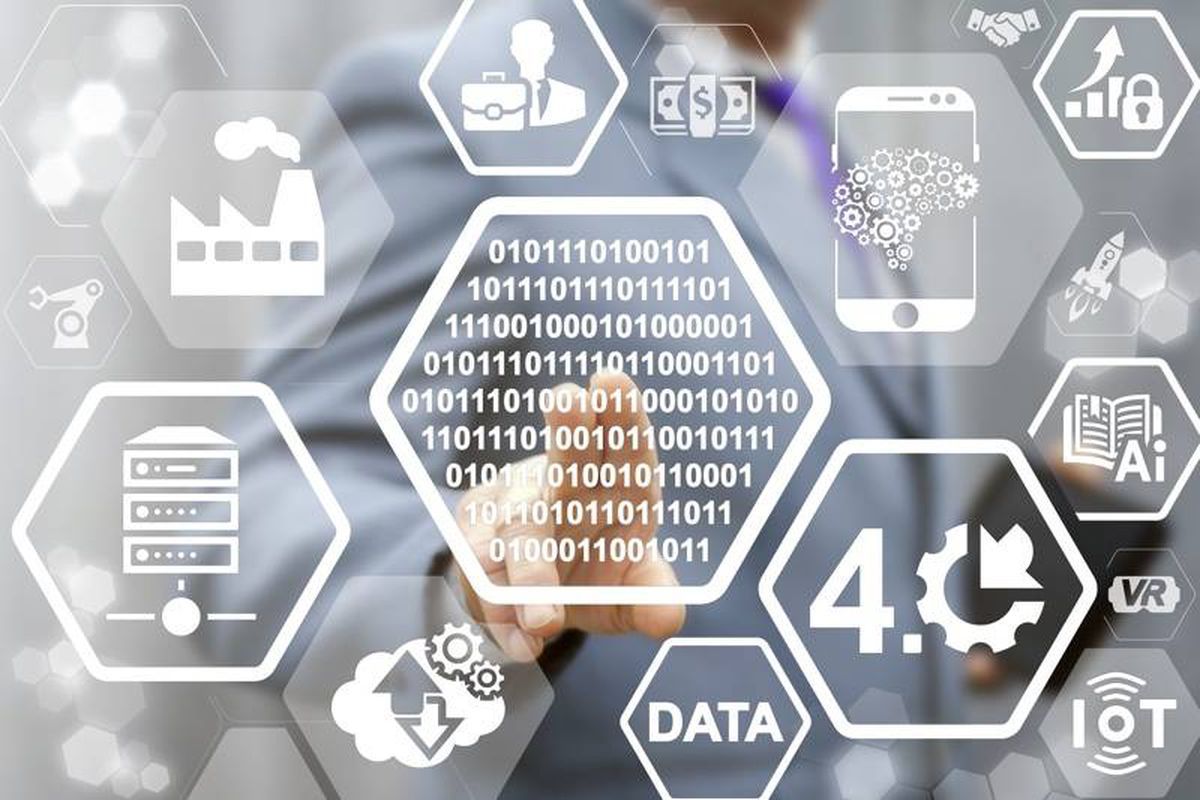
The article was co-authored with Mikhail Pliss, Deputy Director for Expert and Analytical Work at the National Research University Higher School of Economics (HSE).
Introduction
We are currently seeing a great surge of interest in the development and widespread use of artificial intelligence (AI) in medicine and healthcare. It penetrates into a wide variety of areas, such as medical image analysis, healthy lifestyle, communication with patients, recommendations for patient management and treatment, etc. E-health has become almost the main driver of the entire industry as a whole. Rarely do interviews of industry leaders and experts on the topic of reforming and developing healthcare go without comments on computerization, support for medical decision-making and digitalization. These approaches are primarily seen as one of the most effective ways to improve the quality and availability of health care while reducing costs, including reducing ineffective, unnecessary costs, more rational use of resources and new ways of organizing work.
Thanks to a significant step forward in the basic computerization of medical workers, the widespread introduction of electronic health records (EHR), the boom of wearable devices, the active emergence of more and more new methods of examination and treatment based on digital devices, the industry has begun to produce and accumulate a huge amount of information in electronic form. This creates broad prospects in the analysis and use of this information as fuel for launching a wide variety of services and systems based on artificial intelligence, which need it for machine learning and continuous improvement of the accuracy, speed and value of their work [1, 2].
According to a Frost & Sullivan report, healthcare providers and consumers will spend more than $ 6 billion annually on artificial intelligence tools in the coming years. Almost all global IT companies, such as Google, Apple, Facebook, Amazon, Microsoft, Baidu, IBM, Philips, are now investing in this area. The same rise is seen in the sector of startups and specialized private companies that create and begin to offer solutions based on neural networks and machine learning around the world.
In 2017, lots of new methods of using artificial intelligence appeared and in 2018 this growth is only accelerating. Developers are working to improve the efficiency of their solutions, and not only to justify large investments but also in order to gain a foothold in this growing and already extremely competitive market. In 2016 it exceeded $ 320 million in the US and it is predicted to grow at a rate of 38% per year until 2024. The global market for such systems will grow by 39% per year and will reach $ 10 billion by 2024. The main drivers of such growth in healthcare are the increase in treatment costs, the growing number of aging populations and the share of chronic diseases in the total number of medical visits, as well as the imbalance between the number of medical professionals and patients [2, 5].
Russia is trying to seize the moment and ensure leadership in this global trend. For this, at the end of 2016, the Digital Economy project was launched, including the Digital Healthcare industry program. “The Ministry of Health has proposals for the development of the project, including taking into account the opportunities that open up for the use of digital technologies. In particular, there is an idea to develop a clinical decision support system using datasets in the field of medicine, including some approaches to using artificial intelligence. Such a system can help to make a more accurate diagnosis, " claimed Dmitry Medvedev, the Prime Minister, at a meeting of the Presidium of the Council for Strategic Development and Priority Projects in December 2017, http://government.ru/news/30568/
However, despite the massive excitement about artificial intelligence, one should be extremely thoughtful and competent in its application. If we approach the creation of such systems as a fashionable phenomenon, the introduction of "technology for the sake of technology", there are high risks not only to lose the investment in this area but to discredit it in the eyes of the practical healthcare, as well as to grow distrust and rejection in the eyes of patients. In this work, we will highlight the main pitfalls and problems of creating artificial intelligence systems for medicine and healthcare, as well as provide recommendations and considerations for them, based on the study and analysis of trustworthy expert assessments, analytical studies and research publications.
What tasks can be assigned to AI
Andrew Ng from the Google Brain Team and Stanford Artificial Intelligence Laboratory, says that the current media and hype around AI sometimes lends unrealistic power to these technologies. In fact, the real possibilities of using AI are quite limited: modern AI is still capable of giving accurate answers only to simple questions. In his article "What Artificial Intelligence Can and Can't Do Right Now", available at https://hbr.org/2016/11/what -artificial-intelligence-can-and-cant-do-right-now, he explains that the most important thing is to correctly set a task that is feasible for the current level of AI development. As one of the world's recognized AI experts, he concludes that “… surprisingly, despite the wide potential of AI, its real capabilities are still extremely limited. Almost all of the recent advances in AI have come from a situation where some input (A) is used to quickly create a simple answer (B)".
“Therefore, the first and foremost thing that we should consider when planning to create and apply solutions based on AI is a clear wording of the problem for such a system. Andrew Ng offers this recipe for success: “If a typical person can do a mental task in less than one second of thought then we can perhaps automate it using AI now or in the near future."
However, do not flatter yourself with the simplicity and accessibility of this advice. It is simple only in its understanding, but difficult in practical implementation. The problem is that AI can indeed provide an answer to a simple question, but this question should have practical value for the doctor or patient, justifying the costs of creating and the risks of using AI. In other words, we cannot infinitely simplify the formulation of the problem for AI and we do not have to invent it ourselves, at the development of the company, otherwise, we risk creating an unnecessary educational solution that will not have real value in the eyes of medical professionals or patients. When creating products, you need to build on a real-life problem in healthcare that is impossible or very difficult to solve in any other way than with the help of AI.
For example, it hardly makes sense to create a system that would analyze a digital diagnostic image and answer whether it is an X-ray, ultrasound, or not a medical image. This decision is easy enough to make, but what value does it have? When starting the analysis of the data of radiation diagnostics, it is better for us to solve a practical problem or to give an answer to the question that really stands before real doctors. Moreover, this task should be able to be solved by these doctors and its delegation to AI should create some benefit: reduction in medical errors, increase in the speed of analysis, reduction in the cost of providing medical services, increase in the diagnostic or treatment, etc.
Thus, the task of searching for foci of hemorrhage in tomographic images, automatic detection of tumors or other signs (patterns) of pathological processes looks more realistic. Larger-scale tasks, for example, фт automatic analysis of raw diagnostic data and the formulation of a ready-made diagnosis with a computer proposal for further examination and treatment, can be reasonably solved with a hybrid approach, combining not only various artificial intelligence methods that extend beyond popular neural networks and machine learning, but organizational technical means. For example, the identification of symptoms and foci of pathology, as well as image analysis, are really well solved by neural network methods, classification of identified symptoms can be solved using decision trees, probability analysis can be done using conventional methods of statistical processing, searching for and proposing recommendations on patient management tactics may be done using usual algorithmic programming, etc.
In such cases, constructing a solution with a combination of various AI methods, when they are most effective and justified, will have more prospects. By combining individual subtasks with each other, we could accumulate integrated capabilities in systems. This combination can become the key to solving serious problems, allowing you not to idealize AI and not to expect some unprecedented miracles from it and, but at the same time creating products that are in demand on the market and gradually moving forward in the practical application of AI.
A separate large area of promising AI application is the analysis of physician work. Collection of linguistic data contained in the doctor's output information: their appointments, mail, articles, answers to questions, etc. makes it possible to form a semantic cloud of words and concepts that they use, and to determine the drivers and barriers in their motivation, growth, the degree of conservatism of appointments, the level of acceptable risk, and further to form a system of personal tips and individual development plans.
It seems promising to use AI in the field of linguistic services for communicating with patients. The so-called chatbots are text programs that, according to an algorithm, conduct a dialogue with the patient in text communication media (SMS, instant messengers, chats in programs and social networks, etc.), to solve problems most often of a service nature (making an appointment, changing the time of reception, requesting test results, etc.).
At first glance, all this looks too complicated, but one should not get discouraged. In fact, one can and should search for and discuss creative global ideas. It may happen that this idea will not be realistic yet for wide application, but taking advantage of its division into simpler, specialized and feasible tasks, each of which can be solved separately, we will create products with unique capabilities. For example, right now, one can refrain from creating a system that would identify any types of pathologies in any type of diagnostics, narrowing it down to the task of searching for pathologies in some particular type of examination.
Tip number 1. The challenge for AI must come from a real problem, not from technology. It must be within the capabilities of the current level of development of AI methods and tools. It is better to choose a small question or problem that is of practical value for a physician than to invest in a beautiful, but mythical automatic service that claims to replace a medical worker.
Which data is needed to create AI
The Artificial Intelligence for Health and Health Care report, which was commissioned by the Office of the National Health Coordinator (ONC) and the US Agency for Health Research and Quality (AHRQ), was published in December 2017. An advisory group of scientists known as JASON concluded that “…the use of artificial intelligence in medicine and healthcare is indeed promising and feasible. However, the greatest attention needs to be paid to addressing the shortcomings of the massive amounts of data generated by healthcare IT and integrating new data streams that will be critical to the success of AI in healthcare. JASON expressed particular concerns about the quality and availability of relevant data from existing electronic health records (EHR) and the lack of interoperability across the industry” [1].
A very similar thought was expressed by analysts from the UK in their “Thinking on its own: AI in the NHS” report, saying that special attention should be paid “… to the importance of high-quality data for the accuracy of AI algorithms. The quality of the initial data will dictate the quality of the result, as in the saying: "You reap what you sow" [2].
Why is data quality almost the main problem and complexity of creating AI systems? The fact is that almost all known methods of artificial intelligence in one way or another imply the stage of machine learning: the process of computer analysis of pre-prepared data to search for patterns and create the necessary algorithms on their basis, which will then be used to operate the system [3].
There are 3 main methods of machine learning: a) supervised learning, b) reinforcement learning, c) unsupervised learning (self-learning). Analyzing organizational and technical methods, as well as the existing experience in their application, we came to the conclusion that for small companies, start-ups or private developers of medical information systems, the most reasonable choice is supervised learning.
It uses specially selected data in which the correct answers are already known and determined, and the AI parameters are adjusted to minimize the error in its analysis [8]. In this way, the AI can match the correct answers to each input example and reveal possible (including hidden and unknown) dependences of the answer on the input data. For example, a collection of X-ray images with marked pathological foci and coded conclusions will be the basis for teaching an AI, its "teacher". From a series of obtained models, the developer eventually chooses the most suitable one, for example, according to the maximum accuracy of the forecasts.
This approach is attractive primarily for its financial affordability: it is enough to create a small team of developers and experts from several people who have the necessary basic training and provide them with ready-made data consisting of input parameters and correct answers - and such a team will create the necessary prototype of the system in a sufficiently short time. In this case, massive and expensive hiring of doctors, many months of entering data with its labelling, etc. will not be required.
However, machine learning has a great hidden danger, which is connected to the quality of AI work directly depends on the quality of this initial data. In fact, it is not the algorithms, software or hardware of the AI that is its main difficulty, it is the raw data for its training. The principle "garbage in - garbage out", known in classic programming, is even more relevant in AI-based systems.
What is meant by “high-quality” data? We can highlight the following aspects:
- Coverage and a sufficient number of measurements;
- Correctness, accuracy;
- Suitability for machine processing;
- Timeliness;
- Linkedness.
Let's consider the described aspects in more detail.
Coverage and a sufficient number of measurements. The data used to create AI should contain as many combinations of possible human health/disease states as possible. If some phenomenon, say carcinogens, has a significant impact on solving the problem, then the initial data should contain the maximum number of possible variants of such carcinogens and their combination with hundreds of other parameters of human health. If we create a system based on the data of doctors of a certain medical specialty, then the number of doctors present in the sample should be statistically significant: it is not enough to take 500 general practitioners out of several hundred thousand doctors of this specialty working throughout the country.
In some datasets, the collection of information suitable for AI training may not have been foreseen and was not required. This means they can contain a lot of missing data and even errors. This can create problems with the accuracy and objectivity of AI algorithms. In other contexts, data coverage and completeness often concern the representativeness of the sample. This is critical to the accuracy of AI algorithms, as they can be more prone to errors about sub-populations where there is low representation in the sample [1].
A direct consequence of this fact is that in order to create truly reliable AI systems for healthcare, at least hundreds of thousands, or better, millions of examples of raw data are required, such as labelled diagnostic images, structured medical protocols, formalized electronic health records, data from medical devices, etc. The creation and the replication of AI systems based on several hundred or even thousands of data samples should be considered high-risk and immature.
An analysis of projects that have reached a decent level of AI accuracy shows us that a truly gigantic amount of initial data is needed. For example, by building a system that predicts death, discharge, or readmission of a patient who is admitted to hospital, and predicts the final diagnosis, the researchers at Google were able to achieve a truly significant improvement in accuracy over existing prediction systems. But to do this, they had to use 46 billion data points from 216,000 case histories of adult patients. To obtain them, the company entered into an agreement with the medical centers of the University of California at San Francisco and the University of Chicago [8]. The biggest challenge in this type of work, the researchers said, is the sheer volume of difficult-to-handle data contained in health records (EHRs). It is especially difficult to decipher the notes written by doctors by hand [9]. The creation of the IBM Watson system, which was trained for two years, required 605 thousand medical documents from 25 thousand electronic health records taken from the processed giant archive of the Memorial Sloan Kettering Cancer Center in New York to be loaded into the neural network. In addition, 30 billion medical images were analyzed, for which IBM had to buy Merge Healthcare for $ 1 billion. In addition to this process, it was necessary to add 50 million anonymous electronic medical records, which IBM received at its disposal by purchasing the startup Explorys [11].
Correctness, accuracy. If the initial data used in machine learning contains errors in diagnosis and treatment or defective data, then the AI will perceive them as the norm and will itself form incorrect answers. This applies not only to semantic errors but even to spelling errors in text and digital information in all languages in which training is carried out. Therefore, another important requirement is the validation of the data used to create AI. You can't just take and load everything into the training set. It is necessary to have several stages of data verification, including spelling, semantics, completeness, data connectivity and consistency.
Suitability for machine processing. The data that will be used for training must be suitable for computer processing. X-rays should be obtained from digital equipment, not scanned. Human health parameters, for example, blood type, social status, etc., should be presented in the form of generally accepted unified codes, for example, SNOMED CT or at least at the level of the national reference data. Measured parameters should be numerical values, such as height, weight and blood pressure. Where data can be classified and passed as a parameter code = parameter value pair, it should be sent in that form and not as text, HTML layout, or PDF / Word documents. For example, the results of laboratory diagnostics should be transmitted for processing and training of AI in this form, using, for example, the federal laboratory research reference. Only as a last resort, when we really cannot accumulate a sufficient amount of encoded information, it is permissible to store and process it in the form of unstructured text records, for example, complaints, life history data, etc.
It is especially important to transfer information for training in unified formats. Thus, one of the main obstacles to constructing clinical recommendation systems is the difference in the description of date formats, which does not make it possible to arrange the information loaded into the system in a sequence in time, since various systems, doctors of different nationalities and various medical devices encode the date and time in different formats.
Timeliness. The quality of the data can be affected by the timeliness of data entry. All information entered into IT systems is time stamped. These timestamps are used by algorithms in different ways. Therefore, it is important that information is recorded at the time of the event, and not later [1].
Linkedness. When designing an AI system, one should take into account that the health and illness of a patient is a complex multifactorial phenomenon. Even when solving a simple question related to them, a person has to take into account many of the most diverse aspects, each of which, individually at first glance, maybe insignificant. For example, analyzing the results of laboratory diagnostics, the laboratory assistant evaluates not only the objective data of the tests, but can also take into account the characteristics of the reagents, the gender and age of the patient, the presence of chronic pathologies, trauma or stress, heredity, and even the place of residence or work. In this regard, even such a simple question, such as the identification of anemia based on the results of laboratory diagnostics, may consist not only in the analysis of test indicators but also in the combined accounting of other additional data. Therefore, in order for AI to form its conclusion, comparable to what a doctor does, it needs to take into account these additional data.
Thus, in creating truly effective AI systems, it is important to try to ensure data linkedness by loading it from all possible sources that have data consistent with other requirements. For example, when collecting information about laboratory tests, you should also upload social data, anamnesis, data on family relationships and diseases identified in the patient and their relatives, data on their job, education and lifestyle, etc. into the machine learning system. The more related data collected from the patient during the machine learning phase, the higher the potential accuracy of the system.
Compatibility. As we will show below, to create AI it is necessary to use as many different sources of information as possible. For example, when going to analyze information about drug therapy of patients or the results of laboratory tests, it is necessary to collect data from as many medical organizations of various forms of ownership, specialization, and even regions of the location. However, this approach creates a problem of compatibility of these data within the training set. Today, in Russia, the problem of incompatibility of medical data between various software products is very acute. For example, there is still no single reference book of medicines used by the overwhelming majority of medical professionals or a single reference book of laboratory tests. Thus, giving AI as much standardized and machine-readable information as possible can run into the problem of incompatibility of these data. This negated efforts to teach and use AI. Therefore, at the stage of preparing and loading data, it should not only be validated but also reduced to a single classifier/reference book. If there is no such process, you will have to invest in so-called mapping or linking, special processing that forces this data to use a general classifier at the stage of loading into the AI. It is very likely that such procedures cannot be fully automated, in which case it will take time and human manual labor.
Tip number 2. Building a high-quality AI system requires quality data with millions of dimensions. If there is no such data, then one should either make serious investments in its preparation, accumulation and validation (processing), or the risks of successfully creating a product can be extremely large and then one should return to the search for another idea/task that will be provided with the required quantity and quality of the initial data.
Tip number 3. When building an AI system and choosing a data provider, first discuss the quality and standardization of that data with the provider. The sooner this issue is resolved, the less time will be wasted at the stage of launching and piloting the solution.
Where to get data for AI training
Realizing that we need millions of examples of high-quality medical data in order to create AI, the following question arises: where can we get so much data? Are there any sources?
The first answer to this question is the medical information systems of healthcare organizations (MIS), as well as laboratory information systems (LIS) and radiological information systems (RIS / PACS) used in healthcare organizations. This is where patient health records and other electronic medical data are stored in the first place. However, in order to meet all expectations for data quality, AI should be created based on data of many such healthcare organizations, which are located in different regions, with various ways to handle medical documentation, examination and treatment of patients, used equipment and techniques.
If you create AI on the basis of data of a single hospital, even if it is the largest and leading facility in the country, the AI will give the correct answers only within this hospital. The possibility of its replication and its correctness for other healthcare organizations will be questionable or unacceptable at all.
This is exactly the phenomenon that IBM faced, for example, when implementing its cognitive system, IBM Watson. At the stage of replicating the IBM Watson solution in different countries, it turned out that the treatment recommended by this system coincides with the therapy that was prescribed in practice by doctors from medical institutions in the USA, whose medical data were used to create and train its neural network. In USA hospitals, the accuracy of the system reaches 95%. However, for example, in Danish hospitals, doctors conducted their own study of the system, found out that their match rate was only 33% and refused to use the solution. Doctors outside of the United States note that Watson's guidelines do not take national health and medical practices into account. In South Korea, the robot often prescribes treatment that is not covered by the national insurance system, in Taiwan, it is customary to prescribe smaller doses of drugs to prevent side effects [10].
Thus, the system showed its effectiveness only where it was trained because the data used (including medical articles from journals) and all the algorithms of the supercomputer were put in by employees of the American Memorial Sloan Kettering Cancer Center. In addition, this created two problems at once: firstly, not all scientists and doctors in other hospitals, and even more so in countries, agree with the approaches of this research center and not everyone considers it an ultimate authority in oncology. Secondly, in the end, the amount of data that Watson handles turned out to be not as large as it was required. “Suppose you have 10,000 lung cancer patients. This is actually not very much. If there were more, you could see patterns, groups of patients who respond in a certain way or do not respond to therapy, who have certain toxic reactions. This would allow for more personalized and precise medicine. But we cannot do that if we do not have a way to collect this data, ”says Dr Lynda Chin, who installed and trained Watson at MDAnderson Hospital in Texas before she quit her job, and the organization stopped working with IBM [9].
The second most attractive data source is the regional medical information systems (RMIS), in which data is initially collected for the whole subject of the Russian Federation, as well as the services of regional integrated electronic health records and its central node, the federal service of integrated electronic health records from the Unified State Health Information System. In some regions, these sources already contain millions of structured electronic medical documents, and the number of these documents uploaded to the federal service of integrated electronic health records in May 2018 totalled over 460 million records for 70 million patients.
However, there is a problem with these sources too. According to the technical documentation of the federal service of integrated electronic health records, it is allowed to transfer information at the first level of structured electronic medical documents encoding (minimum). Such coding, in fact, means that in the medical documents that were loaded into the service, it was only metadata which were stored in a machine-readable form (which healthcare organization and MIS has transferred the document, date of transfer, medical worker ID, patient ID and several service fields). In addition, the content of the medical document itself could not be transmitted at all (be empty) or be simply transmitted as unmarked text. According to our information, in order to meet the requirements of the Ministry of Health on the terms of integration with the service of integrated electronic health records and the amount of transferred data, many regions were tempted by this opportunity and, as a result, began to collect and transmit data in the structured electronic medical document format No. 1. Such a data source is useless in creating artificial intelligence because the quality of this data does not stand up to scrutiny.
Thus, Regional medical information systems and the service of integrated electronic health records should be considered only if the quality of the data stored in them meets the requirements described above, not only in terms of the number of accumulated records but also in terms of correctness and suitability for machine learning.
Tip number 4. AI training should be based on as many diverse data sources as possible. Only those sources that are stored in a standardized form using international or at least federal reference books and classifiers are subject to use.
Under what conditions the accumulated data can be used
If the developers of the AI system manage to form an interesting and potentially solvable problem and at the same time they find the initial data of sufficient quality and quantity for training, then the following question arises: under what conditions can this data be used?
The problem is that usually some organizations (say, public healthcare facilities) are data operators are, patients are the subjects of this data, and the AI system developer wants to be a consumer of this data, who plans to extract commercial benefits from it. In such a situation, the legal and ethical aspects of data use can become a serious problem.
The first thing you should pay attention to is the transfer of medical information from sources to the system for machine learning, and for processing analysis requests. We believe that such data must be de-identified correctly. This means that the information transmitted to the system does not identify any person and hardly allows a person to be recognized by combination with other data. To meet this requirement, the data does not simply have to not contain the patient's surname, first name and patronymic. There should be no known or identifiable patient identifiers such as personal insurance policy number or passport number, etc. In addition, you should avoid the transfer of information, the use of which could help to identify the patient, for example, the full address of registration / actual residence, the patient's unique code from federal/regional information systems, etc.
Storing information in a depersonalized form is beneficial to developers for several significant reasons at once, including the absence of high requirements for the security class and the absence of undeclared capabilities, the elimination of risks of defamation of the solution in the event of hacker attacks or malicious actions of employees, and a number of others.
Reception and processing of information in a depersonalized form may create the impression that there is no need to obtain the patient's consent since there is no possibility that the data will cause unwanted harm and is personal data. However, we think that not everything is simple here. Yes, on the one hand, there is no explicit legislative requirement (in accordance with Federal Law 152 "On Personal Data" of Russia) to collect such consent. However, this is only a legal view of the situation. From an ethical point of view, everything looks ambiguous. The fact is that during the provision of medical care, patients, of course, agree to the storage and processing of personal data, but only within the framework of that organization and for those purposes that were explicitly indicated in the processing agreement. But after all, this data, albeit in a depersonalized form, will be transferred to "third parties" to create products that have nothing to do with the interests of the patient, and even more so are commercial. In addition, the fact that the operator of such data was not legally restricted in such transfer does not mean that all patients without exception agree to the use of their data for third party benefits.
This problem has yet to be sorted out through public discussions in the expert community. We believe that at this time it is necessary to somehow take into account the interests of patients or society as a whole in such use of data, for example, by providing them for free use for research purposes or creating additional care/services for such patients. If nothing else, operators of such data should not transmit them in secret from the public. Such transfer should be accompanied by the signing of formal agreements, and information about such agreements should be published, or at least provided upon request.
Thirdly, operators of medical data (healthcare and pharmacy organizations, private practitioners, Medical Centers for Information and Analysis, etc.) must understand that they own an essentially priceless treasure that has a direct financial value. In this regard, giving free access to such data to any commercial organization for the creation of their products is at least unreasonable. The use of such data should bring clear benefits to operators, patients and system designers. On the other hand, prohibiting the reuse of already accumulated information means being a dog in the manger and slowing down the emergence of potentially effective solutions and services. One of the ways out of this complex problem is a public-private partnership in various forms. For example, medical organizations could include a clause to the agreement on the storage and processing of personal data that would allow them to use the data in a depersonalized form for the needs of information analysis and the creation of medical solutions. In addition, AI developers could offer these organizations “share and don’t pay” contracts when they use this data, but in return provide employees of such institutions with the created services at no cost.
An example of this approach is the model implemented in the Israeli health insurance organization Clalit (a health insurance fund). Unlike Russian legislation, an Israeli clinic can work with only one insurance fund (insurance company), and, as a rule, works in the IT system of this insurance fund. Thus, Clalit has accumulated over 20 years the data of 5 million Israeli citizens. Currently, the company has launched a project to analyze the collected information and is actively selling a service for changing recommendations for taking medications and their effectiveness, based on many years of verified statistical data to pharmaceutical companies.
However, not all information can be depersonalized. For example, for studies related to quality control of surgical interventions, it is necessary to compare the codes of interventions from the compulsory health insurance system and the data on mortality, disability, and work incapacity certificates from systems of other departments, which can only be done by explicit patient identifiers. It is possible that for research and training of AI in the field of healthcare management and analysis of financial flows in the healthcare system, especially those related to the interaction of funds and insurance companies, it will be necessary to specifically allow the use of personal data of citizens by trusted analytical organizations, where the safety of data of citizens of the Russian Federation is specifically controlled.
Tip number 5. Operators of the accumulated data that will be used to train AI should consider how it can be mutually beneficial for patients and healthcare providers. Developers of AI systems need to think through and formalize the terms and conditions and agreements for the use of this data, drawing up and signing them in the form of legally significant contracts. The list of data sources should be open to the public.
Under what conditions can AI systems be used
Many of the existing AI-based solutions are used today in the direct treatment and diagnostic process, for example, systems for automatic image analysis and pathology detection, chatbots with patients, medical data evaluation systems, etc. At their core, these solutions are medical products. It is believed that from the point of view of certification, they should be subject to the same requirements as for manufacturers of drugs and medical devices.
However, in this matter, not everything is so obvious. On the part of startups and developers of AI services, there are well-founded fears that excessive total regulation will stifle innovation, make product development and release cycles long and expensive, and as a result, we will not only lose technological leadership but also miss a promising opportunity to develop and reduce the cost of medical care [2].
Eleonora Harwich and Kate Laycock, the researchers from the English analytical organization Reform concluded that the creation and implementation of AI systems is a large amount of subjective human labor. Healthcare is a high-risk area where errors can have significant consequences for human life. Therefore, public safety and ethical issues related to the use of AI in healthcare should become the central point of concern for regulatory organizations in this area [2]. The Ministry of Health of the Russian Federation and Roszdravnadzor are such bodies. Perhaps a way out of the situation would be the development by the regulators of a special simplified procedure for verifying the correctness of the system's operation so that people who develop AI algorithms could prove, test and confirm the accuracy and reliability of their algorithms. Now, such verification from the point of view of the current legislation is not provided, therefore, whether to perceive their product as a medical device or not is up to each developer independently.
However, several Russian developers have already faced the fact that AI algorithms published in the open-access based on the open-source model, which are used to solve various problems, incl. problems of health care contain certain errors. The cost of these errors in the operation of AI algorithms in the healthcare sector may turn out to be significant, and so far, the qualifications of domestic developers are sufficient for their timely detection.
Another important aspect of using AI systems is its credibility in the eyes of the medical community. At the moment, there are high risks that the systems created will be met with doubt or even resistance in attempts at mass implementation. Indeed, the excitement surrounding digital healthcare in the media is sometimes a disservice. Technologically ignorant doctors and nurses can get the impression that their industry is being invaded by "IT geeks" whose goal is entertainment or solving far-fetched problems.
For example, in a large survey of the UK public's attitudes towards the use of AI and robotics in healthcare, 47% of respondents noted that "...would like to use an intelligent assistant in healthcare through a smartphone, tablet or PC, a large proportion of them are younger generations. However, when it comes to more sensitive areas, then only 37% said that they would use AI, for example, to monitor the state of the heart, and only 3% would use it to track pregnancy" [4]. The highly-publicized IBM Watson system also faced a problem: “...there is no independent study of it. All articles that talk about its effectiveness were written by the clients of the system; an engineer from IBM is sure to be co-authored. This is not good for modern healthcare” [9]. Michael Hodgkins, Chief Medical Officer of the US AMA, gives examples: “In a recent study of one of the popular [mobile] apps for measuring blood pressure, it was found to be wrong 80% of the time. Another app claimed that it could help identify melanoma from a photo of a mole. In both cases, the [US] Federal Trade Commission stepped in and banned the advertising of these apps because there was no necessary evidence [of the correctness and safety of their work]” [7].
There is a completely understandable explanation for this phenomenon. Healthcare is essentially a very conservative industry and should remain so. The "do no harm" principle is one of the fundamental. Therefore, each new technique, medicine or instrument of the diagnostic and treatment process should be treated with caution and suspicion, asking the question: does this innovation carry unknown risks, hidden defects or direct harm to health?
In this sense, we recommend planning measures to remove such suspicions and prove the usefulness and feasibility of using certain AI-based solutions. AI developers should not just create some new functionality. They must be clearly aware that they are creating products, the results of which will be used in the diagnosis and treatment of real and most often sick people. In addition, this is not just an analysis of some set of numbers. Doctors can perceive them as knowledge and even guidelines for action, which means that such a process must be treated with all the rigor and responsibility, like real scientific medical research. Therefore, developers must prove that their solution improves existing patient management practices and that it is safe. To this end, evidence-based healthcare approaches should be included in the decision-making process. First of all, this is a test of the effectiveness and safety of the proposed solution in clinical trials. Such studies must be carried out in accordance with generally accepted standards. In our country, this is the national standard GOST R 52379-2005 "Good clinical practice", identical to the ICH Harmonized Tripartite Guideline for Good Clinical Practice (ICH GCP) - http://acto-russia.org/index.php?option=com_content&task=view&id=17 (in Russian).
It is necessary to involve authorities in the field of biomedicine and clinical research in the organization of the creation of systems. Their task is to study the created solution or idea and develop a methodology for collecting and analyzing information in such a way that the research results become representative. Such work should include the creation of a clinical trial protocol, a special document that details the purpose, objectives, methods and other aspects of the study.
Tip number 6. The development team should assess whether their solution is a medical device and if so, consider that a complex and costly certification procedure will be required. Clinical trials should be conducted to remove the medical community's suspicions about the safety and effectiveness of the proposed solution. Developers should not rely on the "authority" of medical consultants, no matter how famous they are, as well as publications in the media. Claims about the effectiveness and safety of the service should be based only on correctly conducted experiments, the results of which are published in scientific, peer-reviewed journals, preferably international ones.
However, conducting clinical trials and publishing them is not the only and sufficient measure that developers must consider. Another facet of the peculiarities of medicine is that doctors do not just expect the system to solve a particular problem. It is important for any doctor to understand how the system came to this conclusion. Given the technical features of some AI methods, for example, neural networks and deep machine learning, the created solution can be a "black box", i.e. it can give the doctor quick and correct answers, but sometimes it cannot explain exactly how the system came to this answer and what is the specificity of the technique in this particular clinical situation.
There are AI training scenarios where it is almost impossible for doctors to interpret the results of AI processing. For example, in the Watson Checkup project, medical data were loaded into the system, representing a snapshot of the situation with the incidence in a particular region, after which, as a result of training, the AI computer built correlations of the incidence for one or another characteristic. Sometimes the correlations that AI found could not be explained at the current level of development of medicine (correlations with the length of the arms, the color of the pupils, etc.), but may be explained later, in the course of work on decoding and interpreting the human genome. In such cases, it makes sense to prepare doctors in advance for the fact that the correlation that AI will find in the course of working with data can be used in practice, but cannot be explained theoretically.
From this point of view, the methods used by AI developers should not only be fast and reliable, it is very important for them to be interpretable. However, there is a serious problem in this matter. For example, the same problem can be solved by methods of neural networks, and if there is high-quality initial data for machine learning, such a system can be made with sufficiently high reliability, quickly and cheaply, because no expensive specialists are required. In this case, it will be extremely difficult to explain to the doctor the report issued. On the other hand, if we apply methods that are more suitable for disclosing a hypothesis, say, decision trees or expert systems with the involvement of a person as a teacher, then such systems will be much more expensive to manufacture, but their ability to explain the results of work is much higher.
We recommend that you pay attention to the fact that an information system created with the use of AI should still provide some degree of transparency and deciphering of the results in order to understand how the answer was received, the forecast or this or that recommendation was given. According to Michael Veale, this approach is critical to gaining the trust of healthcare personnel [6]. Systems must have some explanatory power [7].
In actual practice, this means the following: in the system interface, when displaying the analysis results, it is recommended to show a statistical estimate of the probability of a correct decision. If the system uses any clinical guidelines, scientific articles or standards, then citations should be attached to these materials, in ideal with the possibility of online access to them. If the conclusions were based on machine learning methods, then access should be given at least to the description of this training, characteristics and sources of initial data. Of course, this ability to provide explanations is probably technically difficult to achieve in some cases, but developers should nevertheless take this into account in their work.
Tip number 7. You should accompany the output of the AI results with explanations that help the physician interpret the response and understand how the system came to a particular conclusion.
References:
- JASON. Artificial Intelligence for Health and Health Care, December 2018. // URL: https://www.healthit.gov/sites/default/files/jsr-17-task-002_aiforhealthandhealthcare12122017.pdf
- Harwich Eleonora, Laycock Kate. Thinking on its own: AI in the NHS. January 2018. Reform // URL: http://www.reform.uk/publication/thinking-on-its-own-ai-in-the-nhs/
- Richard S. Sutton and Andrew G. Barto. Reinforcement Learning An Introduction - The MIT Press Cambridge, Massachusetts London, England, 2012. – 334 p.
- Hall Wendy, Pesenti Jérôme. Growing the Articial Intelligence Industry in the UK. 2017. // URL: https://www.gov.uk/government/publications/growing-the-artificial-intelligence-industry-in-the-uk
- The Next Generation of Medicine: Artificial Intelligence and Machine Learning. TM Capital Industry Spotlight. 2017. // URL: https://www.tmcapital.com/wp-content/uploads/2017/11/TMCC20AI20Spotlight20-202017.10.2420vF.PDF
- Veale Michael. Logics and Practices of Transparency and Opacity in Real-World Applications of Public Sector Machine Learning, June 2017. // URL: https://arxiv.org/pdf/1706.09249.pdf
- Hodgkins Michael. What’s Missing in the Health Care Tech Revolution. 2017. // URL: http://partners.wsj.com/ama/charting-change/whats-missing-health-care-tech-revolution/
- Gershgorn Dave. Google is using 46 billion data points to predict the medical outcomes of hospital patients. January, 2018. // URL: https://qz.com/1189730/google-is-using-46-billion-data-points-to-predict-the-medical-outcomes-of-hospital-patients/
- Alvin Rajkomar, Eyal Oren, Kai Chen and others. Scalable and accurate deep learning for electronic health records. arXiv:1801.07860v2 [cs.CY] January, 2018. // URL: https://arxiv.org/pdf/1801.07860.pdf
- Alexey Neznanov. Well Interpreted Methods of Data Analysis, February 2018.// URL: https://www.youtube.com/watch?v=5k7KCzEFL4I
- Gusev A.V., Dobridnyuk S.L. Artificial intelligence in medicine and healthcare // Information Society, No. 4-5, 2017. pp. 78-93
- How Dr Watson Couldn't Beat Cancer // URL: http://medportal.ru/mednovosti/news/2017/09/06/879watson/
- IBM pitched its Watson supercomputer as a revolution in cancer care. It’s nowhere close. 2017. // URL: https://www.statnews.com/2017/09/05/watson-ibm-cancer/